AI will enable doctors to be more human
Managing patients with complex needs is often more expensive for healthcare systems that are already strained. It also adds complexity to information flows, as large volumes of healthcare data are no longer primarily available in the hospital. It requires health care systems to shift the focus from episodic care based on admissions to long-term proactive management of chronic care, often in the community or at home. It also requires a different skillset and a strong culture of collaboration between doctors in different specialties, and between doctors and other health practitioners. AI is one of the biggest leverage we have for the creation of this new healthcare perspective.
In this blog article, we’ll review deeply AI and its impact on healthcare:
Artificial intelligence, also known as AI, is a technology that enables machines to produce solutions to complex problems like humans and mimic the way people think. Codes containing 0 and 1, think like you, analyze, and make decisions just like you. In other words, algorithms that mimic the human brain.
Artificial Intelligence (AI) itself is actually the largest and outermost circle in a series of four concentric circles. AI includes many technics inside of it like Machine Learning (ML), Deep Learning (DL), Neural Networks (NN) as shown below.
‘Artificial Neural Networks’ are a common type of machine learning (ML) inspired by the way the human brain works. They progressively improve their ability at a particular task by considering examples. With a large enough data set and powerful enough computer, they will get better and better at this task. They are able to independently find meaningful connections in data.
Although AI is used intertwined with processes such as digitalization, industry 4.0 and automation, AI is only one of the tools that help digitalization and automation. Although methods such as deep learning, machine learning, reinforcement learning are a part of AI, rule-based systems are different from artificial intelligence when we say automation. Rule-based algorithms do not have the capacity to learn from data on their own, they need to be optimized manually.
Anything can be considered Artificial Intelligence if it includes a program that does something that we normally think would rely on a person’s intelligence. The advantages of AI applications are enormous and could revolutionize any professional industry. Let’s see some:
7/24 available
Machines don’t require sleep, frequent breaks, and refreshments like human beings, continuously without getting bored or distracted or even tired.
Multitasking
Machines can think much faster than humans and can perform multi-tasking to obtain the best results
High memory capacity
AI can simultaneously process much more information than humans and discover patterns between them.
Faster Decisions
By using AI alongside other technologies, we can enable machines to make decisions and perform actions faster than a human. When making a decision, the human will analyze many factors both emotionally and practically, but the AI-powered machine works on what it is programmed for and delivers results more quickly.
Enhance creative tasks
AI frees people from routine and repetitive tasks and allows them to devote more time to creative functions.
Provides precision
The application of AI is capable of providing greater precision than humans, for example in industrial environments, machines can make decisions that were previously made manually or monitored without AI.
Explainability
Machine learning algorithms are often described as “black boxes”. Decisions are based on numerous connections between ‘neurons’, they are mostly just mathematically calculations and therefore it is difficult for a person to understand how the result is achieved. This makes it difficult to assess credibility, bias, or detect malicious attacks.
Data requirement
Neural networks need to be trained on large amounts of accurate and reliable data. Incorrect or misrepresentative data can lead to poorly performing systems. Health data are often heterogeneous, complex and poorly coded.
Transferability
The algorithms may be well optimized for the specific tasks they are trained in, but they can be absolutely wrong with data they haven’t seen before.
No Emotions
There is no doubt that the machines are much better when it comes to working efficiently but they cannot get in place of human bond outside of the team. Machines can’t connect with people, which is a feature when it comes for example to Project Management.
Lacking Out of Box Thinking
Machines can only perform the tasks for which they were designed or programmed, anything else could crash or give out irrelevant output that could provide an important basis.
Pattern recognition
Pattern is an entity that can be named, eg. fingerprint image, dermatological image, radiological image, handwritten word, human face, speech signal, DNA sequence and much more. Pattern recognition is a method that examines how machines can distinguish patterns and make some decisions about model categories.
Classification
Classification method is especially aimed at separating two or more groups from each other and finding out which of these classes is most similar to the new data received. The distinction between cat and dog from the classical picture can be given as examples, as well as examples of applications such as the presence or absence of a disease in health, finding the most appropriate from a group of diseases, classifying the severity of the disease.
Natural language processing (NLP)
NLP is one of the AI sub-method that are intensively studied in healthcare, especially for physicians at intensive examinations and visits to easily transfer electronic health records (EHR) to digital with audio narratives and thus speed up their work.
Machine Learning
Machine learning is one of the types of. It enables decision-making from large data sets by finding and processing them. You can view a detailed guide about Machine Learning in Healthcare published by the University of Illinois Chicago.
With the fact that artificial intelligence, especially the image processing part, has found a very common area of influence in the field of health, there has been a very rapid breakthrough in areas where images are important in daily practice. In recent years, many scientific publications and new products have emerged in the fields of pathology, radiology, surgery, cardiology, eye diseases, neurology, and gastroenterology. In addition to image processing, methods such as natural language processing and numerical data analysis found great use in psychiatry and oncology.
You can review the most widely published areas of expertise on artificial intelligence in health from 2010 to the present, from the graph prepared by Bertalan Mesko et al.
We are slowly starting to see real use cases of artificial intelligence in health. So, through which stages will this utilization process become widespread? Let’s take a look together:
Phase 1
Solutions are likely to address the low hanging fruit of routine, repetitive and largely administrative tasks that take up considerable physicians ‘and nurses’ time, optimize healthcare operations and increase adoption. In this initial phase, we will also include imaging-based AI applications currently used in specialist areas such as radiology, pathology and ophthalmology
Phase 2
As patients gain more and more ownership of their care, we expect more AI solutions that support the transition from hospital-based service to home care, such as remote monitoring, AI-powered alert systems or virtual assistants. This phase may include the wider use of NLP solutions in the hospital and home setting, and the use of more AI in multiple specialties such as oncology, cardiology or neurology, where advances are already being made.
Phase 3
In clinical practice, we hope to see more AI solutions in clinical practice with an increasing focus on improved and scaled clinical decision support (CDS) tools in an industry that draws lessons from previous initiatives to introduce such tools to the clinic. Ultimately, participants expect to see AI as an integral part of the healthcare value chain, from how we learn, how we research and deliver care, to how we improve the health of populations. Important prerequisites for AI to deliver its full potential in healthcare will be the integration of larger datasets across organizations, strong governance to continuously improve data quality, and greater trust than organizations, practitioners and patients on the AI systems.
Training and testing on clinically insignificant data
AI needs a well-formed quality dataset for accurate and reliable learning. However, while the data produced due to reasons such as the busy work pace in clinics, documentation, and insurance reimbursement systems requirements, while being accurate for the health system, it can be confusing for AI development.
Lack of independent blind evaluation of real-world data
It is very difficult to obtain data in healthcare, that does not contain any bias and does not vary from person to person, according to expertise and level of knowledge
Narrow applications that cannot be generalized to clinical use
AI models made for specific purposes (for example, bone fracture detection from x-ray images) must include more functions, satisfactory features to can be used in routine practice by the health professionals.
The ‘black box’ nature of neural networks makes it particularly hard to truly assess whether an AI is biased. Worse still, machine learning is very good at identifying proxies for characteristics, such as predicting race and a socioeconomic group from names and postcodes. Because of these problems, the lack of transparency of the process of how an algorithm arrives at the proposed decision poses a major problem for the clinician in assessing whether the diagnosis and recommendation are correct.
Will artificial intelligence make more fair and objective decisions than humans limited by our own personal experiences and prejudices? Or will they collect and even increase human prejudices by placing discrimination in health systems? If the training data is not representative or if the goals are improperly chosen, the resulting AI tool can be extremely unfair. While machine learning algorithms used outside of healthcare have been criticized for discriminating on the basis of race, gender, age, adress, and religion. AI can ‘learn’ false values and even self-actualize – for example, an algorithm to assist in hiring decisions can reward people with the same background as those in historical recruitment data and reinforce bias with every decision.
Replacement or enhancement
AI systems pose significant conceptual challenges for physicians. Most relevant is the controversy between doctors who have replaced by AI or improved with AI.
Current systems for clinical applications can often be regarded as “narrow AI”, meaning they can be successful in “narrow” tasks and can be useful in assisting human physicians with boring, repetitive activities (eg finding elements or compartmentalizing organs or structures in images). As noted, medical professionals interested in the visual analysis of images have begun to experiment with significant changes through such “aids”.
As a result, computerized systems can process many more images than any human operator can achieve better results without the fatigue and time constraints and – if properly trained. Artificial intelligence systems also perform very well in recognizing natural language and written texts; this paves the way for obtaining information from many available sources (eg clinical records) that are often structured, usefully unavailable – formats for systems to learn.
But in general, medical AI systems lack the ability to “interpret” context and “generate” the most distinctive human characteristics (creativity, emotions) and cannot (yet) be considered “powerful” or “generic” AI. Although many questions remain, AI systems can improve many aspects of the doctor’s duties and professionals who choose not to use them will be outdated.
The availability of enormous amounts of data transmitting so many different types of information on each patient requires new professional profiles (not physicians) to discover and extract the most useful items for diagnosis and treatment. The addition of new data sources – in the predictable “expanded personalized medicine” paradigm – will likely require other “medical data scientist” profiles for specific advice for physicians and patients.
AI will enable doctors to be more human
In a time of widespread clinician burnout and staff shortages, AI offers the potential to automate some of the workload and reduce the burden of routine tasks. This can free doctors to engage in more interesting and challenging jobs and offer more flexible working opportunities. Some experts feared that in the long run some experts could be “replaced” by artificial intelligence, which could lead to unemployment, but the breadth of skills and traits required for a doctor cannot be easily duplicated. Artificial intelligence tools that support clinical decision making can enable clinicians to work confidently in a wider area by providing “as needed” access to support from the current pool of information. At the heart of this is an implicit trust in technologies that can be trusted and create tension if there is disagreement or loss of faith. Artificial intelligence can change the type of person who will choose to become a doctor.
In the future, if sophisticated artificial intelligence were to play a dominant role in speaking to patients, processing information and making decisions, this reduction in direct patient interaction and the change in professional roles and tasks could significantly change the everyday nature of health professional.
The strides made in the field of AI in healthcare have been momentous. Moving to a world in which AI can deliver significant, consistent, and global improvements in care will be more challenging. Of course, AI is not a panacea for healthcare systems, and it comes with strings attached. I introduce some strategy that could improve the quality and generality of AI in healthcare:
Working together to deliver quality AI in healthcare
The lack of multidisciplinary development and early involvement of healthcare professionals are among the main obstacles to early tackling new technologies and adopting solutions at scale. One of the problems facing artificial intelligence solutions is to establish clinical evidence of quality and effectiveness. While beginners are interested in scaling solutions quickly, healthcare practitioners should have evidence that any new idea “won’t hurt” before it gets to a patient. Practitioners also want to understand how it works, where the underlying data comes from, and what biases might be embedded in algorithms, so they are interested in crossing the concept of AI as a “black box” to understand what underlies this. Transparency and collaboration between innovators and practitioners will be key to scaling AI in healthcare. User-centered design is another important component of a quality product. Health professionals must be at the center of the design for the end-user, that is, innovation in health.
Rethinking education and skills
We have already touched on the importance of digital skills – these are not part of most practitioners’ arsenal today. AI in healthcare will require leaders well-versed in both biomedical and data science. There have been recent moves to train students in the science where medicine, biology and informatics meet through joint degrees, though this is less prevalent. More broadly, skills such as basic digital literacy, the fundamentals of genomics, AI, and machine learning need to become mainstream for all practitioners, supplemented by critical-thinking skills and the development of a continuous learning mindset. Alongside upgrading clinical training, healthcare systems need to think about the existing workforce and provide ongoing learning, while practitioners need the time and incentive to continue learning.
Strengthening data quality, governance, security, and interoperability
Data access, quality, and availability are potential barriers to the use of AI in healthcare. The data problem is divided into digitizing health to create data, collecting data, and establishing governance around data management. It is critical to ensure basic digitalization of systems and data before embarking on AI deployments – only because the staff’s frustration with basic digitization is leading to a wider AI shift. Healthcare institutions must have robust and compliant data sharing policies that support the care improvements AI offers while providing the right protection measures in a cost-effective manner. The last data issue is that data sets are talking to each other. Policymakers, funding agencies, and nonprofits need to support efforts to adequately anonymize and associate data and, where reasonable, create databases that can be accessed by stakeholders with appropriate safeguards. Healthcare systems need an interconnected data infrastructure to make the most of the rich data available. By providing a prototype for the creation of centers of excellence for AI in healthcare, comprehensive national datasets and networks of innovation clusters or centers can have a significant advantage in terms of their networks and collaborations with academia and industry.
Investing in new talent and creating new roles
Healthcare organizations need to consider how they will develop and recruit new roles that will be critical to the successful introduction and adoption of AI, such as data scientists or data engineers. Demand for such skills is heating up across industries and the competition for talent will be fierce, but many young data professionals find a true vocation in healthcare and its mission and are excited about the potential of digital health and AI. Developing flexible, agile models to attract and retain such talent will be a key part of these organizations’ people strategy.
Funding-Reimbursement
Responsibility for decisions regarding the reimbursement of a drug or device rests with national and local paying organizations, depending on the country, and this decision usually includes what is reimbursed and at what price. Clear criteria for the potential reimbursement of AI applications will be crucial for broad adoption, as well as creative financing models that allow the benefits to be shared among organizations.
The range of applications of AI and AI-mediated technologies in healthcare is broadly and rapidly increasing, with many powerful potential (positive and negative) consequences that can affect people and society at all scales. Many of the headlines I collected in this article remain compelling, as their answers are currently unclear, but our aim must be to open up a multidisciplinary, public discussion of the issues raised to define principles, ethical and social guidelines.
With this basic knowledge of the definition, levels, methods, challenges and potentials of artificial intelligence, we tried to provide an overview of how we can make the medical profession more creative and spend more time with patients than ever before.
If you’d like to explore our startup’s AI based mobile application projects and their potential big impact on healthcare visit our products
Many thanks to Undraw for these beatiful illustrations.
Author
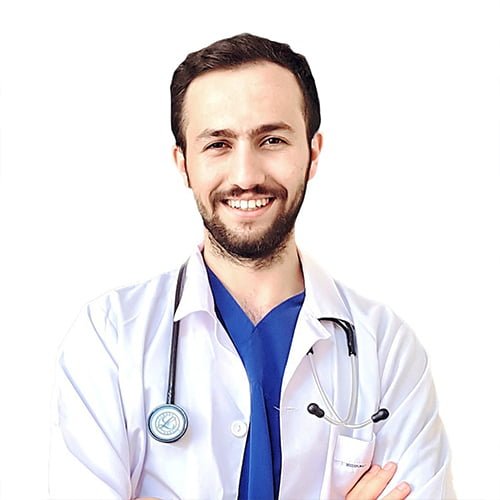
Yusuf Yesil
CEO & Founder
How AI-powered Chatbots are Enhancing Patient Experience - Yesil Science
[…] healthcare is one such sector that can benefit a lot from artificial Intelligence. AI in healthcare is its use at its […]